Reproducing LICO
This project focused on verifying the findings and expanding upon the evaluation and training methods from the paper LICO: Explainable Models with Language-Image Consistency. The main claims are that LICO: enhances interpretability by producing more explainable saliency maps in conjunction with a post-hoc explainability method and improves image classification performance without computational overhead during inference. We reproduced the key experiments conducted by Lei et al. however, the obtained results do not support the original claims....
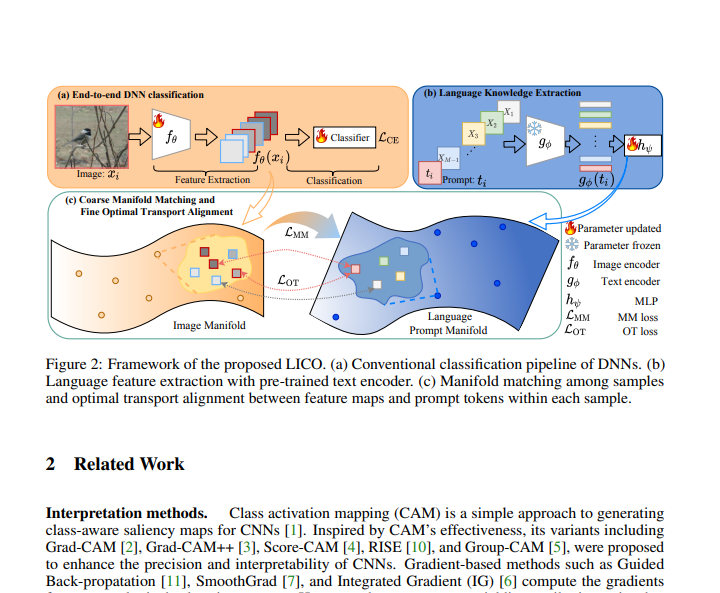